Memo.Translated on May 13, 2025.
“Munson–Rodbard equation is all you need…?”
Several methods exist for calculating the inhibition constant (\(K_{\mathrm i}\)). Besides the widely known Cheng–Prusoff equation, other methods include the Goldstein–Barrett equation, which makes fewer approximations, and the Munson–Rodbard equation, which allows direct calculation using the total concentration of an unlabeled ligand—namely, the 50% inhibitory concentration \({\rm IC}_{50}\)—without requiring approximations. Additionally, the 50% inhibitory concentration of the free ligand, denoted \([I]_{50}\), can be estimated either via nonlinear regression or linear regression.
We investigates the deviation between the estimated \(K_{\rm i}\) values obtained by these methods and the true \(K_{\rm i}\) values using simulated data.
To simulate realistic assay conditions, typical experimental values were used for the binding between the protein kinase C δ-C1B domain (as receptor) and the radiolabeled ligand [³H]phorbol 12,13-dibutyrate ([³H]PDBu):
- Total receptor concentration: \([R_{\rm T}]\) = 3.45 nM
- Total radioligand concentration: \([L_{\rm T}]\) = 17 nM
- Radioligand dissociation constant: \(K_{\rm d}\) = 0.53 nM
For various true values of \(K_{\rm i}\), the ranges of total unlabeled ligand concentration \(\log [I_{\rm T}]\) used in the simulations were:
- \(K_{\rm i}\) = 0.1 nM: from −9.172 to −7.459
- \(K_{\rm i}\) = 1 nM: from −8.454 to −6.496
- \(K_{\rm i}\) = 10 nM: from −7.495 to −5.500
- \(K_{\rm i}\) = 100 nM: from −6.500 to −4.500
(Five data points spaced approximately 0.5 log units apart, centered around the expected 50% inhibition point.)
There was no significant difference between the results of nonlinear regression (fitting to a standard sigmoid function) and linear regression (after logit transformation) in estimating \({\rm IC}_{50}\):
True Ki | [I50] (nM) | True IC50 (nM) | IC50 (nonlinear regression, standard sigmoid) | IC50 (linear regression, logit transformation) |
0.1 nM | 3.02 | 4.75 | 4.71 | 4.68 |
1 nM | 30.2 | 31.9 | 31.9 | 31.9 |
10 nM | 302 | 304 | 303 | 303 |
100 nM | 3019 | 3020 | 3015 | 3014 |
Apparent Ki (nM) | |||||
True Ki | f([IT])a | Cheng–Prusoff | Cheng–Prusoffb | Goldstein–Barrettc | Munson–Rodbard |
0.1 nM | 0.155 | 0.142 | 0.157 | 0.156 | 0.0987 |
1 nM | 1.05 | 0.964 | 1.07 | 1.06 | 1.00 |
10 nM | 10.02 | 9.16 | 10.12 | 10.04 | 9.98 |
100 nM | 99.6 | 91.1 | 101 | 99.8 | 99.8 |
bUsed [L50] in place of [LT] in the Cheng–Prusoff equation. c Approximated \([{\rm I}_{50}]\) with \({\rm IC}_{50}\) in the Goldstein–Barrett equation.
Conclusions
-
The original Cheng–Prusoff equation deviates significantly from the true \(K_{\rm i}\) especially at low values; thus, its use is not recommended.
-
Three methods—(1) nonlinear regression estimating \(K_{\rm i}\) directly, (2) Cheng–Prusoff equation using \([L_{50}]\), and (3) the Goldstein–Barrett equation—all agree with the true \(K_{\rm i}\) value to two significant digits when \(K_{\rm i}\) . Their precision is approximately equal.
-
The Munson–Rodbard equation is consistently accurate across all tested conditions. In particular, when \(K_{\rm i}\) < 1 nM, and the difference between \([I_{50}]\) and \({\rm IC}_{50}\) becomes pronounced, the Munson–Rodbard equation should be used.
Contents
Binding Assay Calculations
The binding assay methodology is based on Sharkey & Blumberg (1985), with modifications. Non-specific binding at equilibrium is neglected. Key equations include:
- Specifin binding: $$\mathbf{[\mathit{RL}_0]} = [\mbox{pellet}_\mbox{(total)}] – k \times [\mbox{supernatant}_\mbox{(total)}] \times \frac{437}{50}$$
Partition Coefficient k: $$\mathbf{\mathit{k}} = \frac{[\mbox{pellet}_\mbox{(nonspecific)}]}{[\mbox{supernatant}_\mbox{(nonspecific)}] \times \frac{437}{50}}$$
Total Ligand Concentration: $$\mathbf{[\mathit{L}_{\mathrm T}]} = [\mbox{pellet}_\mbox{(total)}] + [\mbox{supernatant}_\mbox{(total)}] \times \frac{437}{50}$$
Free Ligand Concentration: $$\mathbf{[\mathit{L}_0]} = [\mbox{supernatant}_{\mbox{(total)}}] = \frac{[L_{\mathrm T}] − [RL_0]}{1 + k}$$
Ligand Concentration at 50% Binding: $$\mathbf{[\mathit{L}_{50}]} = \frac{[L_T] − \frac{[RL_0]}{2}}{1 + k}$$
Total Receptor Concentration: $$\mathbf{[\mathit{R}_\mathrm{T}]} = \frac{K_\mathrm{d} [RL_0]}{[L_0] + [RL_0]}$$
Conversion Factor N from DPM to nM:: $$N = \frac{1}{60} \times \frac{1}{3.7 \times 10^{10}} \times \frac{1}{\mbox{specific activity (Ci/mmol)}} \times \frac{1}{10^3} \times \frac{1}{250 \times 10^{-6}} \times 10^9$$
Non-Specific Binding Considerations
In membrane preparations, non-specific binding can occur due to low-affinity interactions with membrane proteins, phospholipid partitioning, or filter adsorption. These are typically unsaturable and modeled as the product of the non-specific binding coefficient \(k\) and the free radioligand concentration \([L]\) (Hulme and Trevethick, 2010).
In [3H]PDBu binding assays, polyethylene glycol (PEG) is added to precipitate proteins, with γ-globulin included as a co-precipitant, leading to non-specific adsorption (typically \(k = 3–5\%\)). Calculations show that at \(k = 0\), \([RL_0] = 3.321\ \mbox{nM}\) and \([L_0] = 13.7\ \mbox{nM}\); at \(k = 0.04\), \([RL_0] = 3.316\ \mbox{nM}\) and \([L_0] = 13.2\ \mbox{nM}\). High ligand concentrations minimize the impact on bound \([RL]\), allowing correction of free \([L]\) using \(k\).
For unlabeled ligands, if the partition coefficient is assumed equal to that of [3H]PDBu, adjusting the added concentration by dividing by \(1 + k\) provides a good estimate of \([I_\mathrm{T}]\). For example, with a true \(K_\mathrm{i} = 10\ \mbox{nM}\) and \(k = 0.04\), the apparent \(K_\mathrm{i}\) calculated using the Munson–Rodbard equation is \(10.47\ \mbox{nM}\) without correction and \(10.06\ \mbox{nM}\) with correction.
Goldstein–Barrett equation and Cheng–Prusoff equation
Parameters Used
Total Binding Group:
$$[R_0] + [L_0] \rightleftharpoons [RL_0]$$ $$K_{\rm d} = \frac{[R_0][L_0]}{[RL_0]}$$ $$[R_{\rm T}] = [R_0] + [RL_0]$$ $$K_{\rm d} = \frac{([R_{\rm T}] – [RL_0])[L_0]}{[RL_0]}$$ $$[RL_0] = \frac{[R_{\rm T}][L_0]}{K_{\rm d} + [L_0]}$$
Competitive Inhibition Group:
\([I]\): Concentration of free, non-labeled ligand
$$[RI] \rightleftharpoons [I] + [R] + [L] \rightleftharpoons [RL]$$ $$K_{\rm i} = \frac{[R][I]}{[RI]}$$ $$[R_{\rm T}] = [RI] + [R] + [RL]$$ $$[R_{\rm T}] = \frac{[R][I]}{K_{\rm i}} + [R] + [RL]$$ $$[R_{\rm T}] = \left(1+\frac{[I]}{K_{\rm i}}\right)[R] + [RL]$$ $$[R_{\rm T}] = \left(1+\frac{[I]}{K_{\rm i}}\right)\frac{K_{\rm d}[RL]}{[L]}+ [RL]$$ $$[RL] = \frac{[R_{\rm T}][L]}{\left(1+\frac{[I]}{K_{\rm i}}\right)K_{\rm d} + [L]}$$Derivation of the Goldstein–Barrett Equation
At 50% Inhibition of Binding
Let us consider the situation at 50% inhibition of binding: $$\frac{1}{2}[RL_0] = [RL_{50}]$$ $$\frac{1}{2}\frac{[R_{\rm T}][L_0]}{K_{\rm d} + [L_0]} = \frac{[R_{\rm T}][L_{50}]}{\left(1+\frac{[I_{50}]}{K_{\rm i}}\right)K_{\rm d} + [L_{50}]}$$ Multiply both sides and rearrange: $$2\frac{K_{\rm d} }{[L_0]} + 2 = \left(1+\frac{[I_{50}]}{K_{\rm i}}\right)\frac{K_{\rm d} }{[L_{50}]}+ 1$$ $$2\frac{[L_{50}]}{[L_0]} + \frac{[L_{50}]}{K_{\rm d}} = 1+\frac{[I_{50}]}{K_{\rm i}}$$ Solving for \(K_{\rm i}\): $$K_{\rm i} = \frac{[I_{50}]}{2\frac{[L_{50}]}{[L_0]} – 1 + \frac{[L_{50}]}{K_{\rm d}}} \;\;\;\mbox{(Goldstein and Barrett, 1987)}$$ $$= \frac{[I_{50}]}{1 + \frac{[L_{50}]}{K_{\rm d}} + 2\frac{[L_{50}]-[L_0]}{[L_0]}}$$
Conversion to Cheng–Prusoff Equation
If the ligand concentration \([L]\) is sufficiently large compared to receptor concentration \([R]\), then \([L_0] \simeq [L_{50}] \simeq [L_{\rm T}]\), so $$K_{\rm i} = \frac{[I_{50}]}{1 + \frac{[L_{\rm T}]}{K_{\rm d}}}$$.
If the inhibitor concentration \([I]\) is sufficiently large compared to the receptor concentration \([R]\), \([I_{50}]\) can be approximated as the total ligand concentration at 50% inhibition, i.e., \(\rm{IC}_{50}\): $$K_{\rm i} = \frac{\textrm{IC}_{50}}{1+\frac{[L_{\rm T}]}{K_{\rm d}}} \;\;\;\mbox{(Cheng–Prusoff equation)}$$
.Estimating Ki Using Nonlinear Regression
The ideal equation above corresponds to the Hill equation in the case where there is no cooperativity in ligand binding (i.e., \(n=1\)).
Let \(\theta = [RL]/[RL_0]\), then: $$\theta = \frac{[R_{\rm T}][L]}{\left(1+\frac{[I]}{K_{\rm i}}\right)K_{\rm d} + [L]} \times \frac{K_{\rm d} + [L_0]}{[R_{\rm T}][L_0]}$$ $$\theta = \frac{K_{\rm d} + [L_0]}{\frac{K_{\rm d}}{K_{\rm i}}[I] + K_{\rm d} + [L]} \times \frac{[L]}{[L_0]}$$.
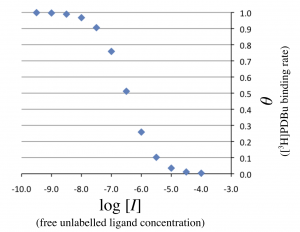
Then, the horizontal axis in Figure 1 is changed to \(\log [I_{\rm T}]\), the logarithmic total concentration of unlabeled ligand.
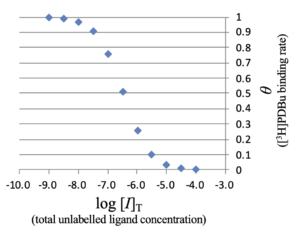
data.csv
logIT,theta -9.495,0.999 -8.995,0.997 -8.495,0.990 -7.995,0.968 -7.496,0.906 -6.996,0.759 -6.498,0.512 -5.999,0.259 -5.500,0.102 -5.000,0.035 -4.500,0.011 -4.000,0.004
Nonlinear Regression Using R
1) \(\theta = f([I])\)
The value of \([L]\) in $$\theta = f([I]) = \frac{K_{\rm d} + [L_0]}{\frac{K_{\rm d}}{K_{\rm i}}[I] + K_{\rm d} + [L]} \times \frac{[L]}{[L_0]}$$ changes as \([I]\) changes. To address this, we substitute the variable \([L]\) with the constant \([L_{50}]\). Also, although \(\theta\) should ideally be a function of the free unlabeled ligand concentration \([I]\), since \([I]\) is unknown, we approximate it using the added total concentration \([I_{\rm T}]\).
> simulation <- read.csv("data.csv") > simulation$IT <- 10^(simulation$logIT + 9) > result1 = nls(theta~(0.53 + 13.679)/(0.53/Ki * IT + 0.53 + 15.339) * 15.339/13.679,data=simulation,start = c(Ki = 10)) > summary(result1)Output:
Formula: theta ~ (0.53 + 13.679)/(0.53/Ki * IT + 0.53 + 15.339) * 15.339/13.679 Parameters: Estimate Std. Error t value Pr(>|t|) Ki 11.0184 0.1833 60.12 3.34e-15 *** --- Signif. codes: 0 ‘***’ 0.001 ‘**’ 0.01 ‘*’ 0.05 ‘.’ 0.1 ‘ ’ 1 Residual standard error: 0.006362 on 11 degrees of freedom Number of iterations to convergence: 4 Achieved convergence tolerance: 1.598e-06
Visualizing the Fit
> plot(theta~IT, data=simulation, log="x") > concpre = seq(-10, -3.5, length=100) > concpre <- 10^(concpre + 9) > lines(concpre, predict(result1, newdata=list(IT=concpre)))
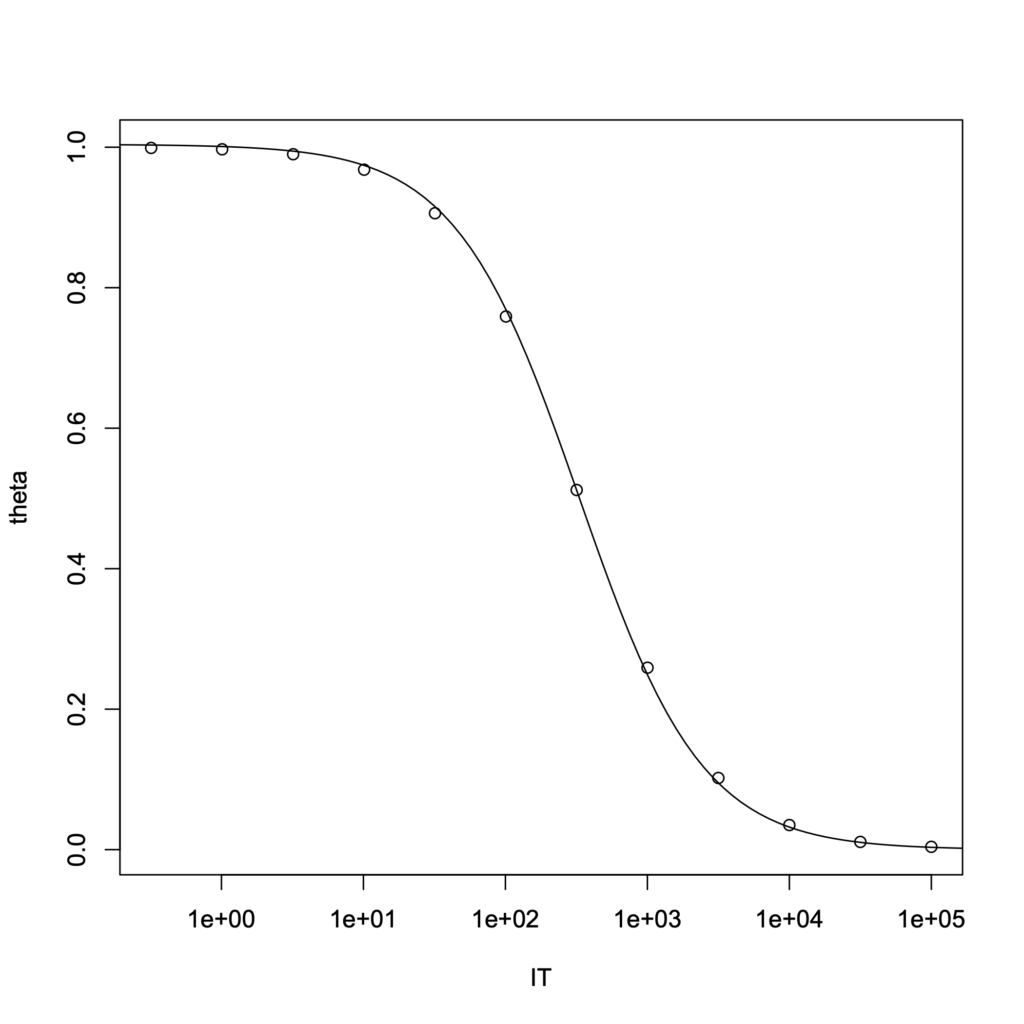
Due to the fixed value of \([L]\), the fit of the curve is not very good. However, the estimated \(K_{\rm i} = 11.0184\ \mbox{nM}\) closely matches the theoretical value (11 nM), indicating that the estimation is quite accurate.
Estimating \({\rm IC}_{50}\)by Regression
Nonlinear Regression
1) Fitting to the Complementary Error Function (erfc)
$${\rm erfc}(x) = \frac{2}{\sqrt{\pi}}\int_{x}^{\infty}e^{-t^{2}}\,dt$$
> simulation <- read.csv("data.csv") > erfc <- function(x) 2 * pnorm(x * sqrt(2), lower=FALSE) > result2 = nls(theta~1/2*erfc((logIT - logIC50)/a), data = simulation, start = c(logIC50=-6.5, a=1)) > summary(result2)
Formula: $$\theta = \frac{1}{2}\cdot\mbox{erfc}\left(\frac{\log[I_{\rm T}] - \log{\rm IC}_{50}}{a}\right)$$
(Note: The complementary error function is multiplied by 1/2 to constrain the range between 0 and 1)
Output:
Formula: theta ~ 1/2 * erfc((logIT - logIC50)/a) Parameters: Estimate Std. Error t value Pr(>|t|) logIC50 -6.476263 0.006851 -945.27 < 2e-16 *** a 1.082295 0.013710 78.94 2.6e-15 *** --- Signif. codes: 0 ‘***’ 0.001 ‘**’ 0.01 ‘*’ 0.05 ‘.’ 0.1 ‘ ’ 1 Residual standard error: 0.005889 on 10 degrees of freedom Number of iterations to convergence: 5 Achieved convergence tolerance: 6.534e-07Visualizing Fit:
> concpre = seq(-10,-3.5, length=100) > plot(theta~logIT,data=simulation,xlim=c(-10,-3.5),ylim=c(0,1)) > lines(concpre, predict(result2, newdata=list(logIT=concpre)))
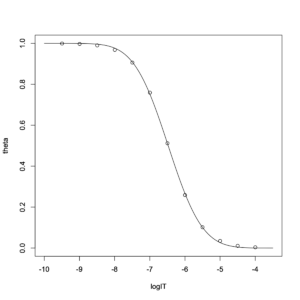
The fit is not very good.
2) Standard Sigmoid Function \(1/(1 + \exp(-x))\):
$$f(x) = \frac{1}{1 + e^{-x}}$$
> sigmoid = function(x) 1/(1+exp(-x)) > result3 = nls(theta~1-sigmoid((logIT - logIC50)/a), data = simulation, start = c(logIC50=-6.5, a=1)) > summary(result3)
Formula: $$\theta = 1 - \frac{1}{1 + e^{-\frac{\log[I_{\rm T}] - \log{\rm IC}_{50}}{a}}}$$
Output:
Formula: theta ~ 1 - sigmoid((logIT - logIC50)/a) Parameters: Estimate Std. Error t value Pr(>|t|) logIC50 -6.476238 0.001243 -5209.7 <2e-16 *** a 0.450384 0.001096 411.1 <2e-16 *** --- Signif. codes: 0 ‘***’ 0.001 ‘**’ 0.01 ‘*’ 0.05 ‘.’ 0.1 ‘ ’ 1 Residual standard error: 0.001071 on 10 degrees of freedom Number of iterations to convergence: 5 Achieved convergence tolerance: 4.447e-07
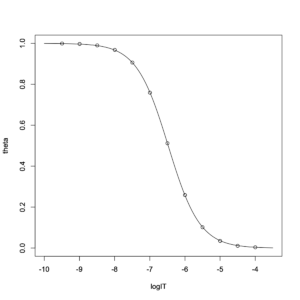
The sigmoid function seems to fit perfectly. This is because, as explained in the linear regression section below, \(\theta\) is related to the logit function, and the standard sigmoid function is the inverse of the logit function.
In practice, the binding ratio \(\theta\) (i.e., [3H]PDBu binding) is usually kept within the range of approximately 0.2–0.8 (20–80%). Thus, using the complementary error function instead of the sigmoid function makes little difference to the results. However, from a modeling perspective, the standard sigmoid function is superior and should be used.
Linear Regression
Next, to visually evaluate the goodness of fit of the fitted curve, a transformation to a linear graph (e.g., for use in Microsoft Excel) is shown.
From the following expression: $$\frac{\theta}{1-\theta} = \frac{\frac{[L]}{[L_0]}(K_{\rm d} + [L])}{\frac{K_{\rm d}}{K_{\rm i}}[I] + K_{\rm d} + [L] - \frac{[L]}{[L_0]}(K_{\rm d} + [L])}$$
Assuming \([L_0] \simeq [L]\), it simplifies to: $$\frac{\theta}{1-\theta} = \frac{K_{\rm d} + [L]}{\frac{K_{\rm d}}{K_{\rm i}}[I]}$$
Taking the logarithm (base 10): $$\log \left(\frac{\theta}{1-\theta}\right) = -\log [I] -\log K_{\rm d} + \log K_{\rm i} + \log (K_{\rm d} + [L])$$ This results in a linear relationship with a slope of \(-1\) when plotted against \(\log[I]\) (free ligand concentration) (logit function) (Figure 3). (Note: \([L]\) on the right-hand side also depends on \([I]\), so this is an approximation.)
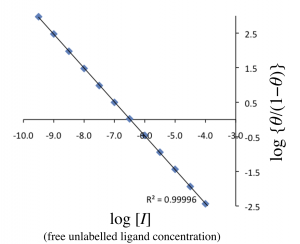
Although the horizontal axis in the figure above is \(\log[I]\) (free unlabeled ligand), this value is not experimentally accessible. Instead, we use the added total ligand concentration \(\log[I_{\rm T}]\), and obtain:
- \(\log{\rm IC}_{50} = -6.4765\) from the linear plot
- \(\log{\rm IC}_{50} = -6.4762\) from nonlinear regression using the standard sigmoid function.
- 333.8 nM (from linear regression),
- 334.0 nM (from nonlinear regression).
Simple Conversion from Binding Ratio to \(K_{\rm i}\)
For weak ligands that do not inhibit binding by more than 50%, \(K_{\rm i}\) can be calculated from a single data point using the following rearranged form: $$\frac{\theta}{1-\theta} = \frac{K_{\rm d} + [L]}{\frac{K_{\rm d}}{K_{\rm i}}[I]} \rightarrow K_{\rm i} = \left(\frac{\theta}{1-\theta}\right) \frac{K_{\rm d}[I]}{K_{\rm d} + [L]}$$ If the unlabeled ligand concentration \([I]\) is sufficiently large compared to the receptor concentration \([R]\) (which is the case for weak ligands), \([I]\) can be approximated by the total ligand concentration \([I_{\rm T}]\) $$K_{\rm i} = \left(\frac{\theta}{1-\theta}\right) \frac{K_{\rm d}[I_{\rm T}]}{K_{\rm d} + [L]}$$
Munson–Rodbard Equation
When the concentration of the inhibitor \([I]\) is not sufficiently high relative to the receptor concentration \([R]\) — for instance, when the binding affinity of the unlabeled ligand is equal to or greater than that of the labeled ligand PDBu — it is not appropriate to approximate \([I]\) with the total ligand concentration \([I_{\rm T}]\). In such cases, the Munson–Rodbard correction allows you to calculate \(K_{\rm i}\) using the actual \({\rm IC}_{50}\) (total concentration), without relying on the approximation \([I_{50}] \simeq \textrm{IC}_{50}\) (Munson and Rodbard, 1988; Huang, 2003).
If we define \(y_0 = [RL_0]/[L_0]\), then: $$K_{\rm i} = \frac{\textrm{IC}_{50}}{1+ \frac{[L_{\rm T}] (y_0 + 2)}{2 K_{\rm d} (y_0 + 1)} + y_0} - K_{\rm d} \left(\frac{y_0}{y_0 + 2}\right) \;\;\;\;\mbox{(Eq.26; Munson–Rodbard equation) }$$
When \(y_0\) is very small, this equation reduces to the Cheng–Prusoff equation.
Derivation of the Munson–Rodbard Equation
Let \(K_1 = 1/K_{\rm d}\), \(K_2 = 1/K_{\rm i}\). Then: $$[RL] = K_1 [R] [L]\;\;\;\;\mbox{(Eq.27)}$$ $$[RI] = K_2 [R] [I]\;\;\;\;\mbox{(Eq.28)}$$ From the conservation laws: $$[L_{\rm T}] = [RL] + [L]\;\;\;\;\mbox{(Eq.29)}$$ $$[I_{\rm T}] = [RI] + [I]\;\;\;\;\mbox{(Eq.30)}$$ $$[R_{\rm T}] = [RI] + [R] + [RL] = K_2 [R] [I] + [R] + K_1 [R] [L] = [R] (1+ K_1[L] + K_2 [I])\;\;\;\;\mbox{(Eq.31)}$$
Now consider the conditions without inhibitor and at 50% inhibition.
Let the ratio without inhibitor be defined as \(y_0 = [RL_0]/[L_0]\). From Eq.29: $$[L_{\rm T}] = [L_0] (1 + y_0)\;\;\;\;\mbox{(Eq.32)}$$ $$[L_0] =\frac{[L_{\rm T}]}{1 + y_0}\;\;\;\;\mbox{(Eq.33)}$$ $$[RL_0] = \frac{ y_0 [L_{\rm T}]}{1 + y_0}\;\;\;\;\mbox{(Eq.34)}$$
At 50% inhibition: $$[RL_{50}] = \frac{y_0 [L_{\rm T}]}{2 (1 + y_0)}\;\;\;\;\mbox{(Eq.35)}$$ From Eq.29: $$[L_{50}] = [L_{\rm T}] - [RL_{50}] = \frac{ [L_{\rm T}] (2 + y_0) }{2 (1 + y_0)}\;\;\;\;\mbox{(Eq.36)}$$
Using Eq.27, the free receptor at 50% inhibition is: $$[R_{50}] = \frac{[RL_{50}]}{K_1 [L_{50}]} = \frac{y_0}{K_1 (y_0 + 2)}\;\;\;\;\mbox{(Eq.37)}$$
From Eq.28 and Eq.30: $$\textrm{IC}_{50} = [I_{{\rm T} 50}] = [RI_{50}] + [I_{50}] = [I_{50}](1+K_2 [R_{50}])\;\;\;\;\mbox{(Eq.38)}$$ Substituting Eq.37 into Eq.38: $$[I_{50}] = \frac{\textrm{IC}_{50}}{1+\left(\frac{K_2}{K_1}\right)\left(\frac{y_0}{y_0+2}\right)}\;\;\;\;\mbox{(Eq.39)}$$ Solving Eq.31 for \([R]\) and substituting into Eq.27: $$[RL] = \frac{K_1 [R_{\rm T}] [L] }{1+ K_1 [L] + K_2 [I]}\;\;\;\;\mbox{(Eq.40)}$$
Without inhibitor, using Eq.34 and Eq.33: $$[R_{\rm T}] = y_0 \left(\frac{1}{K_1} + \frac{[L_{\rm T}]}{1 + y_0}\right)\;\;\;\;\mbox{(Eq.41)}$$
At 50% inhibition: $$[RL_{50}] = \frac{K_1 [R_{\rm T}] [L_{50}] }{1+ K_1 [L_{50}] + K_2 [I_{50}]}\;\;\;\;\mbox{(Eq.42)}$$
Solving Eq.42 for \(1/K_2\): $$\frac{1}{K_2} = \frac{[I_{50}]}{\frac{K_1[R_{\rm T}][L_{50}]}{[RL_{50}]} - K_1[L_{50}] - 1}$$
Substitute Eq.39 into this and simplify: $$\frac{1}{K_2} + \frac{1}{K_1}\left(\frac{y_0}{y_0+2}\right) = \frac{\mathrm{IC}_{50}}{\frac{K_1[R_{\rm T}][L_{50}]}{[RL_{50}]} - K_1[L_{50}] - 1}$$
Now, substitute:
- Eq.36 into \([L_{50}]\)
- Eq.41 into \([R_{\rm T}]\)
- Use \([L_{50}][RL_{50}] = \frac{(y_0 + 2)}{2y_0}\)
Therefore: $$\frac{1}{K_2} = \frac{\textrm{IC}_{50}}{1+ \frac{[L_{\rm T}] (y_0 + 2)}{2 \left(\frac{1}{K_1}\right) (y_0 + 1)} + y_0} - \left(\frac{1}{K_1}\right) \left(\frac{y_0}{y_0 + 2}\right)\;\;\;\;\mbox{(Eq.43)}$$
Finally, replacing \(1/K_2 = K_{\rm i}\), \(1/K_1 = K_{\rm d}\), we recover Eq.26.
Dandliker's Equation
You can calculate \(K_{\rm i}\) from each data point in an inhibition experiment (Dandliker, 1981).
Define: $$\chi = \frac{[RL]}{[L]}$$ (Note: \([L]\) is computed as \([L] = [L_{\rm T}] - [RL]\))
Then: $$K_i = \frac{[I_{\rm T}] K_d \chi}{[R_{\rm T}] - \frac{[L_{\rm T}]\chi}{1 + \chi} - K_d \chi} - K_d \chi\;\;\;\;\mbox{(Eq.44)}$$
Also, define \(f_b = [RL]/[L_{\rm T}]\)/ Since, \(\chi = f_b/(1-f_b)\), then: $$K_{\rm i} = \frac{[I_{\rm T}] K_{\rm d} f_b}{[R_{\rm T}](1 - f_b) - K_{\rm d} f_b - [L_{\rm T}] f_b (1 - f_b)} - \frac{K_{\rm d} f_b}{ 1 - f_b}\;\;\;\;\mbox{(Eq.45)}$$
Eq.45 is a rearranged form of Equation (11) in Huang, 2003.
Derivation of Dandliker’s Equation
As before, define \(K_1 = 1/K_{\rm d}\), \(K_2 = 1/K_{\rm i}\). $$\chi = \frac{[RL]}{[L]} = K_1 ([R_{\rm T}] - [RL] - [RI])\;\;\;\;\mbox{(Eq.46)}$$ $$\frac{[RI]}{[I]} = K_2 ([R_{\rm T}] - [RL] - [RI])\;\;\;\;\mbox{(Eq.47)}$$ $$[L_{\rm T}] = [RL] + [L]\;\;\;\;\mbox{(Eq.48)}$$ $$[I_{\rm T}] = [RI] + [I]\;\;\;\;\mbox{(Eq.49)}$$ $$[RL] = \frac{[L_{\rm T}]\chi}{1 + \chi}\;\;\;\;\mbox{(Eq.50)}$$ $$[L] = \frac{[L_{\rm T}]}{1 + \chi}\;\;\;\;\mbox{(Eq.51)}$$ From Eq.46, express \([RL]\) and \([L]\) in terms of \(\chi\): $$[RI] = [R_{\rm T}] - [RL] - \frac{\chi}{K_1} = [R_{\rm T}] - \frac{[L_{\rm T}]\chi}{1 + \chi} - \frac{\chi}{K_1}\;\;\;\;\mbox{(Eq.52)}$$
Solving Eq.47 for \([I]\): $$[I] = \frac{[RI]}{K_2} \times \frac{1}{[R_{\rm T}] - [RL] - [RI]}\;\;\;\;\mbox{(Eq.53)}$$ Since \([RI] = [R_{\rm T}] - [RL] - \frac{\chi}{K_1}\), then: $$[I] = \frac{[R_{\rm T}] - [RL] - \frac{\chi}{K_1}}{K_2} \times \frac{1}{\frac{\chi}{K_1}} = \frac{K_1}{K_2\chi}\left([R_{\rm T}] - \frac{[L_{\rm T}]\chi}{1 + \chi} - \frac{\chi}{K_1}\right)\;\;\;\;\mbox{(Eq.54)}$$ Substitute Eqs. 52 and 54 into Eq.49: $$[I_{\rm T}] = \left(1 + \frac{K_1}{K_2\chi}\right) \left([R_{\rm T}] - \frac{[L_{\rm T}]\chi}{1 + \chi} - \frac{\chi}{K_1}\right)\;\;\;\;\mbox{(Eq.55)}$$
Solve Eq.55 for \(1/K_2\), giving: $$K_i = \frac{1}{K_2} = \frac{[I_{\rm T}] K_d \chi}{[R_{\rm T}] - \frac{[L_{\rm T}]\chi}{1 + \chi} - K_d \chi} - K_d \chi\;\;\;\;\mbox{(Eq.56)}$$
References
- Sharkey, N. A.; Blumberg, P. M. Highly lipophilic phorbol esters as inhibitors of specific [3H]phorbol 12,13-dibutyrate binding. Cancer Res. 1985, 45, 19–24. [URL] [PMID]: 3855281.
- Dandliker, W. B.; Hsu, M.-L.; Levin, J.; Rao, R. Equilibrium and kinetic inhibition assays based upon fluorescence polarization. Methods Enzymol. 1981, 74, 3–28. DOI: 10.1016/0076-6879(81)74003-5. PMID: 7321886.
- Goldstein, A.; Barrett, R. W. Ligand dissociation constants from competition binding assays: errors associated with ligand depletion. Mol. Pharmacol. 1987, 31, 603–609. [URL] PMID: 3600604.
- Hulme, X. C.; Trevethick, M. A. Ligand binding assays at equilibrium: validation and interpretation. Br. J. Pharmacol. 2010, 161, 1219–1237. DOI: 10.1111/j.1476-5381.2009.00604. PMID: 20132208.
- Huang, X. Equilibrium competition binding assay: inhibition mechanism from a single dose response. J. Ther. Biol. 2003, 225, 369–376. DOI: 10.1016/S0022-5193(03)00265-0. PMID: 14604589.
- Munson, P. J.; Rodbard, D. An exact correction to the Cheng-Prusoff correction. J. Receptor Res. 1988, 8, 533–546. DOI: 10.3109/10799898809049010. PMID: 3385692.